Why can Reinforcement Learning achieve results beyond Optimal Control in many real-world robotics control tasks?
Why can Reinforcement Learning achieve results beyond Optimal Control in many real-world robotics control tasks?
A central question in robotics is how to design a control system for an agile, mobile robot. This paper studies this question systematically, focusing on a c...
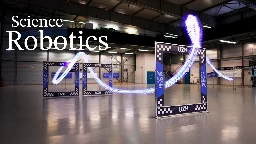
RL's most impressive achievements are beyond the reach of existing optimal control-based systems. However, less attention has been paid to the systematic study of fundamental factors that have led to the success of reinforcement learning or have limited optimal control. This question can be investigated along the optimization method and the optimization objective. Our results indicate that RL outperforms OC because it optimizes a better objective: OC decomposes the problem into planning and control with an explicit intermediate representation, such as a trajectory, that serves as an interface. This decomposition limits the range of behaviors that can be expressed by the controller, leading to inferior control performance when facing unmodeled effects. In contrast, RL can directly optimize a task-level objective and can leverage domain randomization to cope with model uncertainty, allowing the discovery of more robust control responses. This work is a significant milestone in agile robotics and sheds light on the pivotal roles of RL and OC in robot control.
Source: Davide Scaramuzza